Our upcoming magazine issue is devoted to long-term problems. Few problems are longer-term or more intractable than America’s systemic racial inequality. And a particularly entrenched form of it is housing discrimination.
A long history of policies by banks, insurance companies, and real estate brokers has denied people of color a fair shot at homeownership, concentrated wealth and property in the hands of white people and communities, and perpetuated de facto segregation. Though these policies—with names like redlining, blockbusting, racial zoning, restrictive covenants, and racial steering—are no longer legal, their consequences persist, and they are sometimes still practiced covertly or inadvertently.
Technology has in some cases exacerbated America’s systemic racial bias. Algorithmically based facial recognition, predictive policing, and sentencing and bail decisions, for example, have been shown to consistently produce worse results for Black people. In housing, too, recent research from the University of California, Berkeley, showed that an AI-based mortgage lending system charged Black and Hispanic borrowers higher rates than white people for the same loans.
Could technology be used to help mitigate the bias in housing instead? We brought together some experts to discuss the possibilities. They are:
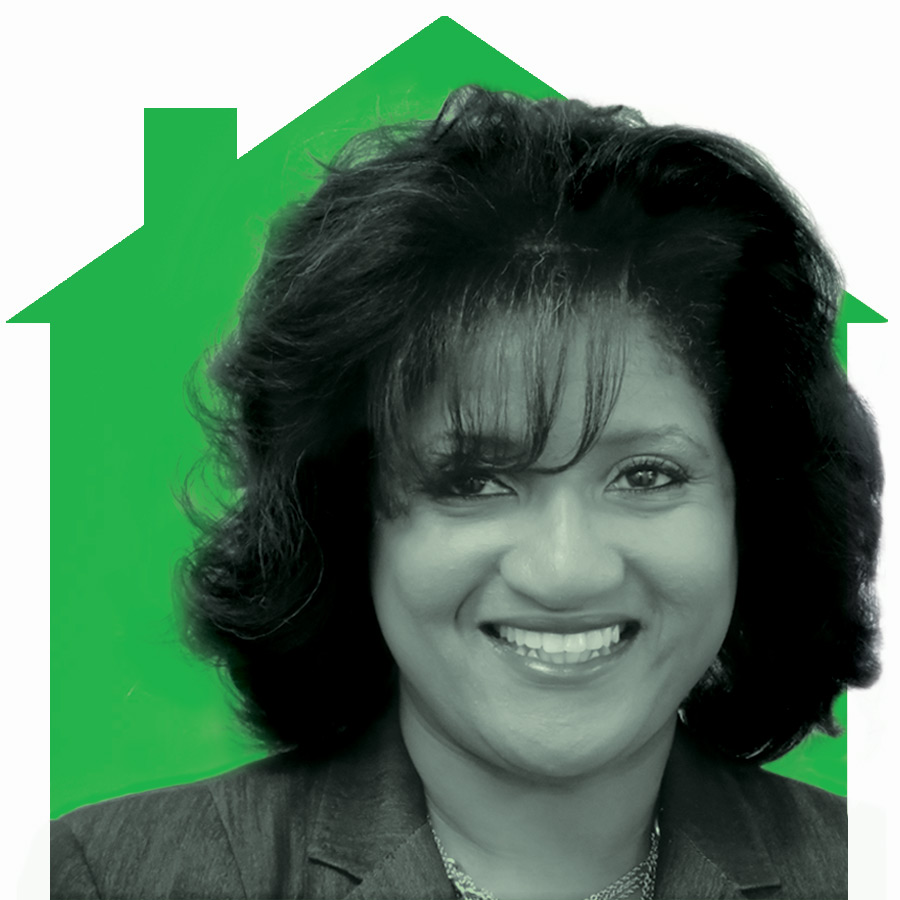
Lisa Rice
President and CEO of the National Fair Housing Alliance, the largest consortium of organizations dedicated to ending housing discrimination.
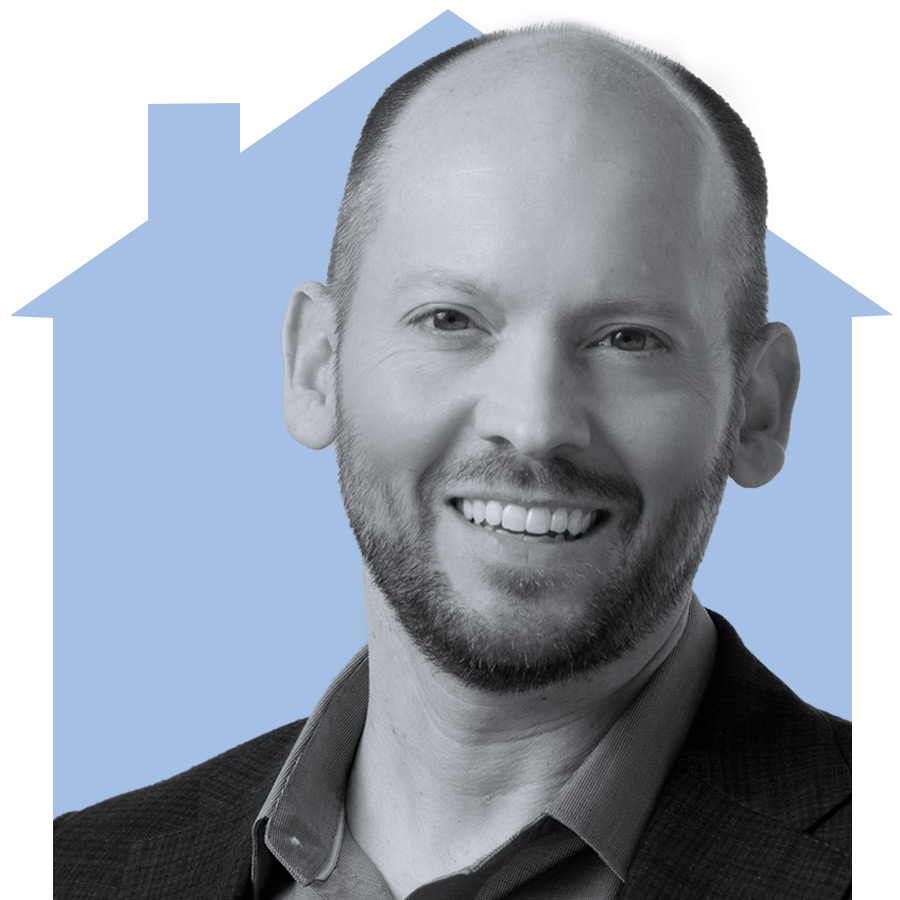
Bobby Bartlett
Law professor at UC Berkeley who led the research providing some of the first large-scale evidence for how artificial intelligence creates discrimination in mortgage lending.
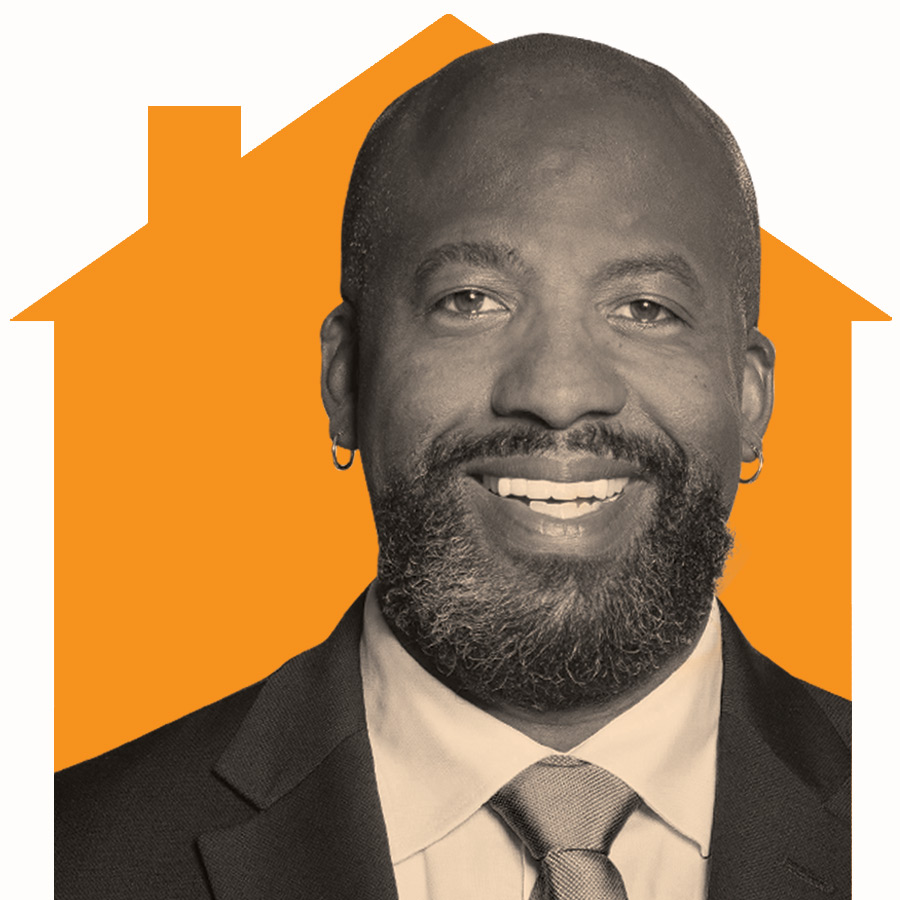
Charlton McIlwain
Professor of media, culture, and communication at NYU and author of Black Software: The Internet & Racial Justice, from the Afronet to Black Lives Matter.
This discussion has been edited and condensed for clarity.
McIlwain: When I testified before Congress last December about the impact of automation and AI in the financial services industry, I cited a recent study that found that unlike human loan officers, automated mortgage lending systems fairly approved home loans, without discriminating based on race. However, the automated systems still charge Black and Hispanic borrowers significantly higher prices for those loans.
This makes me skeptical that AI can or will do any better than humans. Bobby—this was your study. Did you draw the same conclusions?
Bartlett: We had access to a data set that allowed us to identify the lender of record and whether that lender used a totally automated system, without any human intervention—at least in terms of the approval and underwriting. We had information on the race and ethnicity of the borrower of record and were able to identify whether or not the pricing of approved loans differed by race. In fact, it did, by roughly $800 million a year.
Why is it the case that these algorithms, which are blinded to the race or ethnicity of the borrower, would discriminate in this fashion? Our working hypothesis is that the algorithms are often simply trying to maximize price. Presumably, whoever is designing the algorithm is unaware of the racial consequence of this single-minded focus on profitability. But they need to understand that there is this racial dynamic, that the proxy variables they’re using—in all likelihood, that’s where the discrimination is. In some sense, there’s effectively redlining of the reddest sort going in through the code. It resembles what happens in the mortgage market generally. We know that brokers will quote higher prices to minority borrowers, knowing that some will turn it away, but others will be more likely to accept it fora whole host of reasons.
McIlwain: I have a theory that one of the reasons that we end up with biased systems—even when they were built to be less discriminatory—is because the people designing them don’t really understand the underlying complexity of the problem. There seems to me to be a certain naïveté in thinking that a system would be bias free just because it is “race blind.”
Rice: You know, Charlton, we had the same perspective that you did back in the ’90s and early 2000s. We forbade financial institutions from using insurance scoring, risk-based pricing, or credit scoring systems, for just this purpose. We realized that the systems themselves were manifesting bias. But then we started saying you can use them only if they help people, expand access, or generate fairer pricing.
McIlwain: Do people designing these systems go wrong because they really don’t fundamentally understand the underlying problem with housing discrimination? And does your source of optimism come from the fact that you and organizations like yours do understand that complexity?
Rice: We are a civil rights organization. That’s what we are. We do all of our work through a racial equity lens. We are an antiracism organization.
In the course of resolving redlining and reverse redlining cases, we encouraged the financial institutions and insurance agencies to rethink their business models, to rethink how they were marketing, to rethink their underwriting guidelines, to rethink the products that they were developing. And I think the reason we were able to do that is because we are a civil rights agency.
We start by helping corporations understand the history of housing and finance in the United States and how all of our housing and finance policies have been exacted through a racial lens. You can’t start at ground zero in terms of developing a system and think that system is going to be fair. You have to develop it in a way that utilizes antiracist technologies and methodologies.
McIlwain: Can we still realistically make a dent in this problem using the technological tools at our disposal? If so, where do we start?
Rice: Yes—once the 2008 financial crisis was over a little bit and we looked up, it was like the technology had overtaken us. And so we decided, maybe if we can’t beat it, maybe we’ll join. So we spent a lot of time trying to learn how algorithmic-based systems work, how AI works, and we actually have come to the point where we think we can now use technology to help diminish discriminatory outcomes.
If we understand how these systems manifest bias, we can get in the innards, hopefully, and then de-bias those systems, and build new systems that infuse the de-biasing techniques within them.
We really don’t have regulatory agencies who understand how to conduct an exam of a lending institution to ferret out whether or not its system is biased.
But when you think about how far behind the curve we are, it’s really daunting to think about all the work that needs to be done, all the research that needs to be done. We need more Bobbys of the world. But also all of the education that needs to be done so that data scientists understand these issues.
Rice: We’re trying to get regulators to understand how systems manifest bias. You know, we really don’t have a body of examiners at regulatory agencies who understand how to conduct an exam of a lending institution to ferret out whether or not its system—its automated underwriting system, its marketing system, its servicing system—is biased. But the institutions themselves develop their own organizational policies that can help.
The other thing that we have to do is really increase diversity in the tech space. We have to get more students from various backgrounds into STEM fields and into the tech space to help enact change. I can think of a number of examples where just having a person of color on the team made a profound difference in terms of increasing the fairness of the technology that was being developed.
McIlwain: What role does policy play? I get the sense that in the same way that civil rights organizations were behind the industry in terms of understanding how algorithmic systems work, many of our policymakers are behind the curve. I don’t know how much faith I would place in their ability to realistically serve as an effective check on the system, or on the new AI systems’ quickly making their way into the mortgage arena.
McIlwain: I remain skeptical. For now, for me, the magnitude of the problem still far exceeds both our collective human will and the capabilities of our technology. Bobby, do you think technology can ever help
this problem?
Bartlett: I have to answer that with the lawyerly “It depends.” What we see, at least in the lending context, is that you can eliminate the source of bias and discrimination that you observed with face-to-face interactions through some sort of algorithmic decision making. The flip side is that if improperly implemented, you could end up with a decision-making apparatus that is as bad as a redlining regime. So it really depends on the execution, the type of technology, and the care with which it is deployed. But a fair lending regime that is operationalized through automated decision making? I think that’s a really challenging proposition. And I think that jury is still out.
from MIT Technology Review https://ift.tt/34gwuyx
No comments:
Post a Comment